Usecase UMC Utrecht
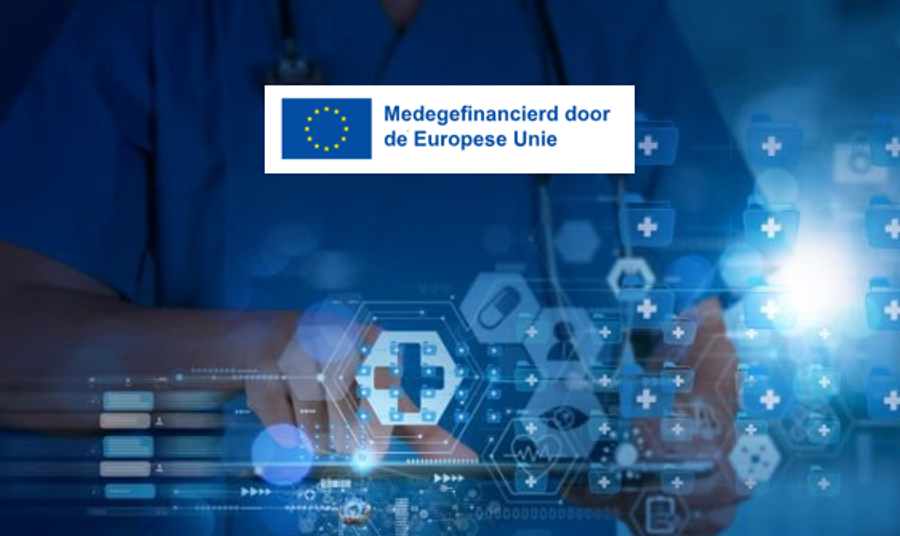
Challenge
Pancreatic cancer is one of the most lethal forms of cancer, with only 9 out of 100 patients still alive after 5 years. The optimal treatment for pancreatic cancer consists of surgery combined with chemotherapy. Of the approximately 2,500 people who are diagnosed with pancreatic cancer in the Netherlands each year, about 500 patients can undergo surgery; for the remaining patients, the cancer is locally inoperable or the disease has already metastasized. However, for all patients who can undergo surgery, the disease returns in 80% of the patients within 2 years: this is called a recurrence. A disease recurrence is generally determined with CT scans. A radiologist assesses whether tumor growth is visible on these scans. Often, after surgery, it is very difficult to distinguish tumor tissue around the site of the operation from scarring of the tissue. UMC Utrecht, in collaboration with Datacation, wants to employ Artificial Intelligence to diagnose recurring pancreatic cancer with greater certainty at an early stage.
Process
This large, challenging project is divided into multiple phases, with various AI models being developed that complement each other well. This way, we quickly achieve the first tangible results, and our work can grow with the latest developments in the field. Our first AI solution is an innovative computer vision model that can recognize and segment the pancreas in a CT scan. During an operation, the tumor and part of the pancreas are removed from the patient. As a result, the pancreas looks different on the post-operative CT scan for everyone. Existing segmentation models work suboptimally because of this variation. Team Datacation has developed an innovative segmentation model based on an encoder-decoder neural network with an attention mechanism. This work has resulted in a scientific publication. Currently, Team Datacation is developing AI that can detect whether a tumor has returned after surgery. With the help of a powerful semi-supervised classification model, a distinction can be made between CT scans with recurring tumors and CT scans without tumors. To support the doctors and make the classification explainable, segmentation and attention are used to distinguish between scar tissue (fibrosis) and tumors.
Solution
UMC Utrecht and Datacation currenlty work closely together on this project. The ultimate goal is to eventually integrate the AI model into clinical practice, where it will serve as support for radiologists. As a result, patients are expected to gain clarity more quickly about the presence of a recurrence, and the likelihood of recurrence treatment increases, with the aim of improving survival and quality of life for patients.